GIS and artificial intelligence: what is GeoAI?
GeoAI refers to the integration of artificial intelligence techniques and technologies with geospatial data and analysis. It combines the capabilities of AI with geographic information systems (GIS) to extract meaningful insights from spatial data.
According to a report by IndustryARC, the AI for geospatial analytics market is expected to grow to $172 million by 2026, highlighting the enormous potential and importance of this trend.
In this article, we explore the significance of geospatial artificial intelligence, its core technologies, and its synergies with GIS, highlighting the importance of fully exploiting GeoAI’s capabilities and its transformative impact on GIS enterprises. We also discuss the best implementation strategies and the barriers that need to be overcome.
The importance of geospatial artificial intelligence in GIS industry
GeoAI plays a significant role in the geographic information systems industry by using advanced algorithms and methods to extract key insights from spatial data. Its integration with GIS systems brings many benefits that improve decision-making and resource management. At its core, GeoAI enhances spatial data analysis by leveraging machine learning techniques to recognise complex patterns, relationships and anomalies in geographic data sets. This analytical knowledge promotes a comprehensive understanding of spatial dynamics, enabling stakeholders in diverse areas such as urban planning, environmental monitoring, disaster management, agriculture and transportation to make informed decisions.
Geospatial AI streamlines GIS data processing and analysis tasks through automation. Automating labour-intensive processes reduces the time and resources required for manual analysis, thereby increasing productivity. In turn, this efficiency allows GIS professionals to focus on interpreting results and developing strategies based on the insights gained from the data, therefore increasing productivity. GeoAI enhances predictive modelling and forecasting capabilities by using historical geospatial data to identify trends and patterns with greater accuracy. It also enables monitoring and response in real-time through the continuous analysis of streaming geospatial data. These systems can quickly detect changes or anomalies in the environment and trigger automatic responses or alerts, particularly valuable in many areas, e.g. smart cities. Here, GeoAI monitors traffic patterns, air quality and infrastructure health to optimise resource allocation and enhance public safety.
Key technologies empowering GeoAI
Machine Learning is a fundamental pillar that uses algorithms to analyse and interpret geospatial data. It enables GeoAI systems to learn patterns and relationships in geospatial data, enabling predictive modelling, classification and task grouping. These algorithms can be applied to different types of geospatial data (multi- and hyperspectral satellite imagery, GPS data and geotagged social media data).
Deep learning excels at analysing complex and unstructured geospatial data, such as satellite and aerial imagery multitemporal data-cubes and cloud points. These models can automatically extract features and patterns from large geospatial datasets for specific tasks.
Computer vision enables the interpretation and analysis of visual geospatial data such as satellite and aerial imagery. Advanced computer vision algorithms can identify and classify features, land cover types, infrastructure and changes over time from images, enabling applications such as land use mapping, disaster response and urban planning.
Natural language processing techniques facilitate the analysis of unstructured geospatial text data, as well as the extraction of geospatial information, sentiment analysis and object recognition from text data, enabling applications such as geospatial trend analysis, disaster monitoring and opinion-based urban planning.
Remote sensing uses a variety of sensors on satellites and aircraft to collect data about the Earth’s surface, atmosphere and oceans, providing comprehensive perspectives for geographic analysis and monitoring.
Big data and cloud computing provide the infrastructure needed to efficiently handle massive amounts of geospatial data, enabling scalable processing and storage capabilities.
The Internet of Things (IoT) devices equipped with sensors, GPS receivers and communication modules generate huge amounts of geospatial data related to environmental conditions, infrastructure performance and human activities. GeoAI integrates IoT data streams for applications, enabling data-driven decisions and proactive interventions.
Foundation models are a class of deep learning models pre-trained on large amounts of diverse data across a wide range of tasks, giving them a broad understanding of the world. These models, such as Segment Anything, Presto or Prithvi-100M, serve as a foundation upon which specific models can be trained further for specialised tasks. The key feature of foundation models is their ability to use extensive pre-training to adapt to different tasks with minimal additional training data, making them incredibly efficient. This contrasts with the traditional ML approach, where models are typically trained from scratch for each task.
How can LLM and GenAI help you draw valuable conclusions and recommended actions?
Large language models (LLM) and generative artificial intelligence (GenAI) can make it much easier to understand maps, draw conclusions and recommend actions thanks to advanced language processing capabilities. LLMs can comprehensively analyse data presented on maps, identifying patterns, relationships and trends in the information. In turn, GenAI can generate meaningful insights and conclusions based on knowledge that incorporates relevant geospatial mapping findings, ensuring a clear understanding of the implications and meaning of the mapped data.
By integrating LLMs with vector storage solutions and 3rd party APIs, GeoAI applications can also efficiently access, extract and synthesise relevant content from vast datasets. This integration allows for the dynamic interpretation of geospatial data, enabling the models to generate insights based on real-time and historical data. For instance, when a user queries about environmental changes in a specific area of interest, LLMs can quickly process the query, recognise the intent, and pull relevant geospatial information from 3rd party API and vector storages to fetch the latest environmental reports. The model can then summarise this information, providing the user with a comprehensive answer. By leveraging LLMs, GeoAI is set to transform how we interact with and understand geospatial information, making complex geographical analysis more accessible than ever before.
LLM and GenAI enable enterprises to extract valuable insights from geospatial data by discovering hidden insights, identifying spatial relationships, and predicting future outcomes. These insights can help inform many decisions, from optimising transportation routes and urban development to mitigating environmental risks, planning for natural disasters, and more.
The most impactful AI use cases in geospatial and GIS industry
As technology advances, there is a growing awareness of its transformative potential: artificial intelligence algorithms combined with the analytical capabilities of GIS are revolutionising the way spatial data is used in countless fields. The combination of artificial intelligence and GIS holds great promise for better understanding spatial phenomena, optimising the use of resources and supporting more informed decision making. This combination not only provides organisations with practical knowledge, but also opens new opportunities for innovation and sustainability.
There are several ways in which artificial intelligence can be used in the GIS industry:
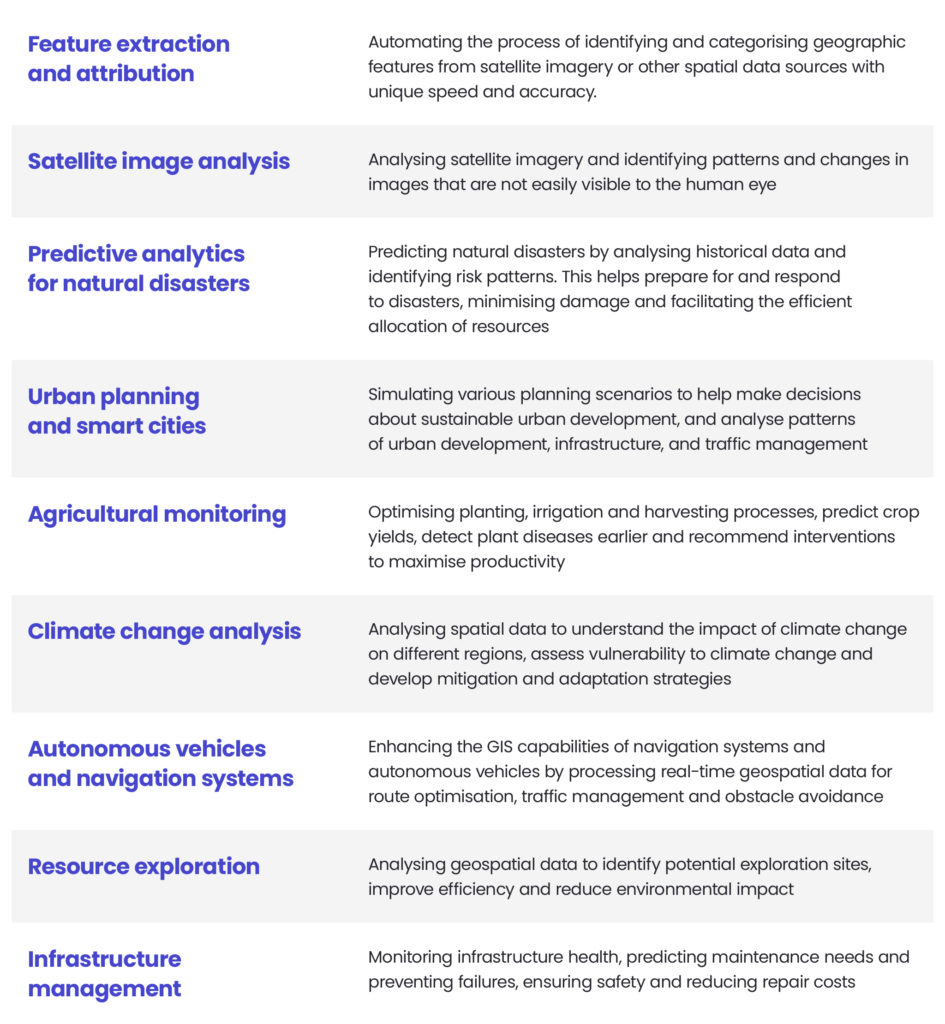
Benefits of GeoAI adoption for your GIS company
Increased efficiency and productivity
By using AI algorithms, GIS businesses are able to process greater volumes of geospatial data in less time, leading to increased efficiency and productivity. This efficiency allows employees to focus on more complex tasks that require human expertise, ultimately improving overall service delivery.
Enhanced accuracy and precision in spatial analysis
GeoAI algorithms are capable of analysing vast amounts of geospatial data with greater accuracy and precision than traditional methods. By integrating AI techniques such as machine learning and computer vision, GIS companies can extract valuable insights from complex datasets, leading to more accurate spatial analysis and decision-making. This increased accuracy enables customers to make informed decisions based on reliable geospatial information, increasing the value of GIS services.
Cost savings through automation and optimisation
By automating repetitive tasks and optimising workflows with GeoAI, GIS organisations can achieve significant savings. They are able to reduce overhead costs associated with data processing, analysis and project management, reducing manual labour and increasing operational efficiency. These savings can make geospatial services more accessible and affordable while improving profit margins for GIS organisations.
Competitive advantage in the market
GIS companies using GeoAI offer their clients advanced capabilities and innovative solutions based on artificial intelligence for spatial analysis, automation and optimisation. The ability to deliver higher quality services in less time, with greater accuracy and efficiency, positions GIS companies as leaders in the geospatial industry, ultimately driving growth and success in the marketplace.
Challenges and limitations
Real-time satellite data processing
Satellite imagery is often subject to atmospheric interference or distortion, which can affect accurate analysis. The sheer volume of data generated by satellites requires efficient processing methods and robust algorithms to extract meaningful insights.
Data quality
Data quality is a significant concern in GeoAI applications. Low-cost geospatial data often comes with low resolution, which may limit the accuracy of AI models trained on such data. Conversely, high-resolution data can be prohibitively expensive and pose challenges regarding storage, processing power, and computational resources required for analysis. The consistency, inaccuracies, and biases in the data used to train AI models can undermine the reliability and effectiveness of GeoAI systems.
Technology complexity
Technology integration in GeoAI implementation is a significant challenge. It involves combining different technologies such as GIS platforms, AI algorithms, remote sensing techniques and geospatial databases. Coordinating these components is complex and requires expertise in both geospatial analysis and artificial intelligence development. Computing requirements add another layer of complexity, as processing geospatial data and training AI models requires significant computing resources, especially for large datasets or complex algorithms.
Weather and cloud cover
Weather conditions can have a significant impact on the quality and availability of geospatial data, particularly satellite imagery. Cloud cover can reduce visibility and prevent accurate data collection, which can affect the performance of GeoAI algorithms that rely on such data. Adverse weather conditions can limit the deployment and operation of aerial drones or other remote sensing technologies, further complicating data collection and analysis processes in geospatial applications.
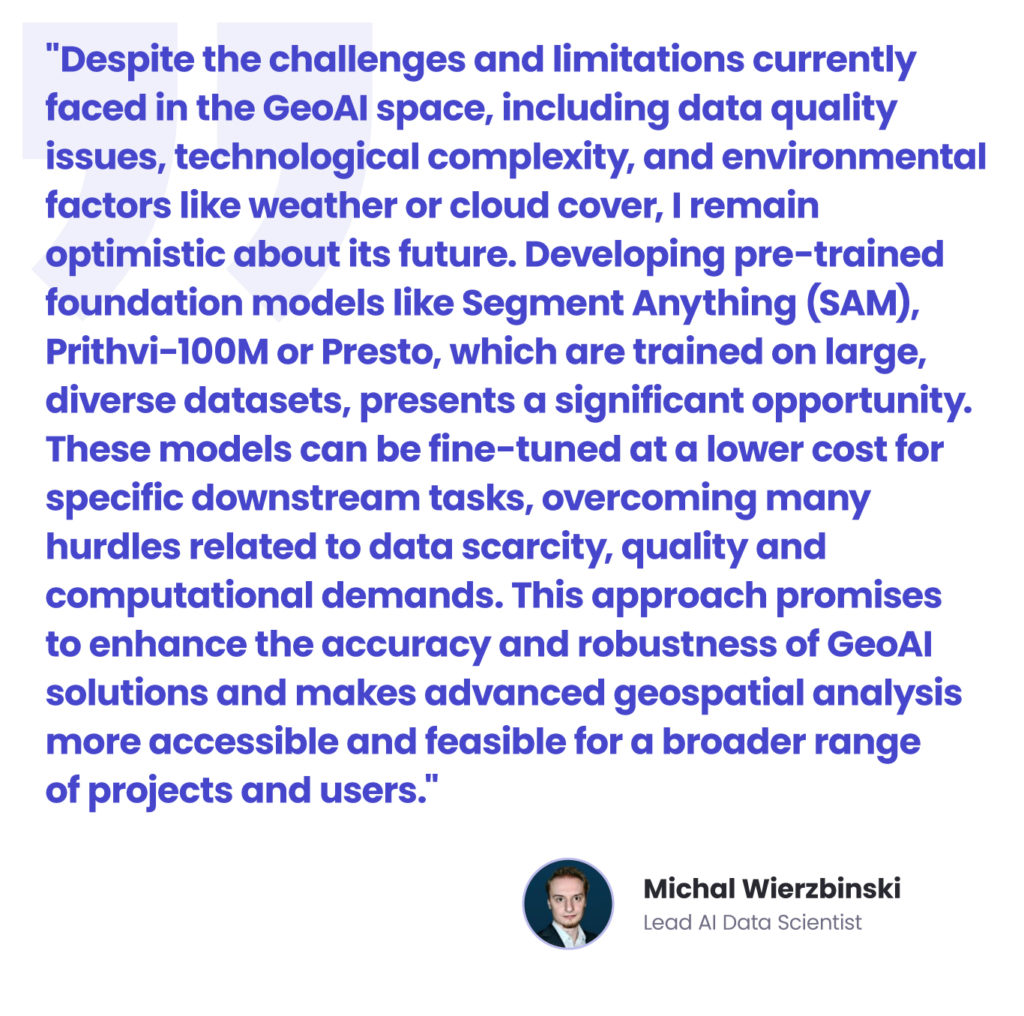
See how we leverage AI technology in geospatial solutions to drive better results
Smart City Analytics with Talent Alpha
We worked with Talent Alpha, a pioneering company in transforming the future of work, to improve their Digital Twin model for a large city using cutting-edge artificial intelligence techniques. The focus was on predictive analytics in public transport, addressing the key challenges of predicting a number of Bus Entries and modelling arrival times of the buses.
The cooperation began with detailed data diagnostics, defining the scope of predictions and exploring additional data sources. We’ve developed customised machine learning models using techniques ranging from time series analysis to advanced algorithms such as XGBoost and MLP. The initial proof of concept showed significant improvements over the baseline models, particularly in predicting passenger entry within 15 minutes.
We also delivered a comprehensive data pipeline to model bus arrivals accurately, including real-time data ingestion, data cleaning, and custom algorithms. Despite limited datasets, the predictive model demonstrated accuracy comparable to GPS-based live systems, with improved performance observed for longer distances between buses and stations.
In addition to predictive capabilities, we also provided insightful analyses using interactive visualisations and anomaly prediction.
Development of events classification system
We started collaborating with Ordnance Survey Leisure because they needed a solution to filter and select suitable events and activities for their outdoor activities. We customised an event classifier to solve their problem using pre-trained NLP and transfer learning models. By using a relatively small data sample, we achieved ~95% classification accuracy.
Throughout the project, we worked closely with the client to develop a mutual understanding of the business problem and the available data landscape. By collaborating at every project lifecycle stage, we created a system that reflected our client’s business needs.
Azure AI architecture design
Our client was working on an R&D project to monitor open-cast mines, including their productivity and environmental impact, and planning new mining operations and machine telematics. They required a sophisticated solution capable of analysing extensive satellite imagery and using artificial intelligence to provide informed recommendations and predictive insights to achieve these goals.
Our team set about developing an innovative artificial intelligence cloud architecture within the Microsoft Azure environment. This architecture spans the entire lifecycle of AI products, from seamless data ingestion to robust data storage, powerful analytics and rigorous AI model training, publishing and deployment. Embracing the best practices of MLOps, we prioritised increased efficiency through workflow optimisation and extensive automation.
The resulting platform is scalable, ensuring seamless expansion as the number of monitored mines grows. In addition, its flexibility facilitates the training and management of multiple AI models tailored to different use cases. The platform successfully supports over 10 AI models in active production, demonstrating its adaptability and reliability in real-world scenarios.
The areas in which GeoAI can be used are almost endless, and its benefits can be seen in both business and day-to-day life. So, if you need help with the implementation of artificial intelligence solutions in your GIS company feel free to reach out to our expert by filling up the form below!
About the author
Contact us
Let’s get in touch to check how we could support your project.
RECOMMENDED READING