AI in geospatial: exploring the capabilities of ChatGPT as a use case generation tool (part 2)
Welcome back to the second instalment in our exciting blog series, where we dive into the world of use case generation with ChatGPT for various industries. After a thrilling start with ChatGPT’s brainstorming capabilities on AI in automotive: exploring the Capabilities of ChatGPT as a use case generation tool (part 1), we are now setting our sights on the geospatial industry.
Geospatial technology is nothing short of magical; it provides us with the tools to visualise, measure, and analyse the Earth’s features, empowering decision-makers and transforming industries as varied as agriculture, logistics, and disaster management. The integration of Artificial Intelligence into geospatial technology brings a new era of solutions and services.
In this article, we’ll be leveraging ChatGPT to generate a medley of innovative ideas tailored for the geospatial industry. We aim to explore how the blend of large language models and geospatial data can contribute to smarter, more efficient, and sustainable solutions in various domains. Similar to our previous article, the content generated by ChatGPT will remain unaltered, and we will interlace it with our insights, perspectives, and experiences to shed light on the practicality and ingenuity of the ideas.
Generating use-cases for Geospatial industry
Title: Transforming the Geospatial Industry: AI Use Cases for Computer Vision, Text Analytics, NLP, Machine Learning, and Recommendation Engines
Introduction:
Artificial Intelligence (AI) has emerged as a game-changer in various industries, and the geospatial sector is no exception. Leveraging cutting-edge technologies such as computer vision, text analytics and natural language processing (NLP), machine learning, and recommendation engines, AI is revolutionizing how geospatial data is collected, analyzed, and utilized. In this article, we will explore the diverse applications of AI in the geospatial industry, highlighting four to five examples for each technology area.
AI-Powered Computer Vision:
Computer vision technology enables machines to interpret and understand visual data, providing valuable insights for the geospatial industry. Here are some notable AI use cases:
- Object Detection and Recognition: AI algorithms can accurately identify and classify objects within geospatial imagery, enabling applications like automatic vehicle counting, infrastructure inspection, and land cover analysis.
- Image Segmentation: AI-based image segmentation allows precise identification and extraction of specific features within geospatial images, enabling applications such as urban planning, vegetation analysis, and flood mapping.
- Change Detection: By analyzing historical and current satellite imagery, AI can detect changes over time, aiding in environmental monitoring, disaster response, and urban growth analysis.
- Defect Detection: AI algorithms can analyze geospatial images to identify defects in infrastructure, such as cracks in roads or buildings, enabling efficient maintenance and asset management.
- Anomaly Detection: Leveraging computer vision, AI can detect anomalies in geospatial data, helping identify irregularities such as illegal activities, encroachments, or abnormal traffic patterns.
Text Analytics and NLP:
Text analytics and natural language processing techniques enable the geospatial industry to extract valuable insights from unstructured textual data. Consider the following AI use cases:
- Geospatial Data Extraction: NLP algorithms can extract location-specific information from text sources such as social media feeds, news articles, or customer reviews, facilitating geospatial analysis and decision-making.
- Sentiment Analysis: AI-powered sentiment analysis can evaluate public sentiment towards specific locations or events, aiding in urban planning, tourism management, and public opinion monitoring.
- Named Entity Recognition: NLP techniques can identify and extract specific entities like place names, addresses, or landmarks from unstructured text, enabling geocoding, geotagging, and location-based services.
- Language Translation: AI-powered language translation allows real-time translation of multilingual data, facilitating cross-cultural communication and analysis of global geospatial information.
- Geospatial Query Processing: NLP-based query processing systems enable users to extract geospatial information by asking natural language questions, improving the accessibility and usability of geospatial data.
Machine Learning:
Machine learning algorithms enable geospatial data analysis, pattern recognition, and prediction. Here are some compelling AI use cases within the geospatial industry:
- Geospatial Predictive Analytics: By leveraging historical geospatial data, machine learning models can predict outcomes like traffic congestion, disease outbreaks, or land use changes, aiding in proactive decision-making.
- Spatial Clustering: Machine learning algorithms can identify spatial clusters within geospatial datasets, helping to uncover patterns, hotspot detection, and optimizing resource allocation.
- Geospatial Data Fusion: Machine learning techniques can integrate heterogeneous geospatial datasets from multiple sources, facilitating data harmonization, spatial interpolation, and better decision support.
- Geospatial Image Classification: Machine learning models can classify satellite or aerial imagery into different land cover types, enabling applications such as environmental monitoring, precision agriculture, and forestry management.
- Geospatial Data Compression: Machine learning algorithms can optimize the storage and transmission of geospatial data by identifying patterns and reducing redundancy, leading to more efficient data management and faster data processing.
Recommendation Engines:
Recommendation engines leverage AI to provide personalized recommendations based on user preferences and geospatial data. Here are some notable applications within the geospatial industry:
- Location-Based Recommendations: AI-powered recommendation engines can suggest points of interest, restaurants, tourist attractions, or relevant services based on a user’s location, preferences, and historical data.
- Route Optimization: By analyzing geospatial data and user preferences, recommendation engines can provide optimal routes for navigation, considering factors like traffic congestion, road conditions, and user preferences for scenic or efficient routes.
- Personalized Travel Planning: Recommendation engines can suggest customized travel itineraries, considering a user’s interests, time constraints, and geospatial data, enhancing the travel planning experience.
- Land Parcel Selection: AI-driven recommendation engines can assist in land parcel selection for specific purposes like real estate development, infrastructure projects, or conservation efforts, considering factors such as soil quality, accessibility, and environmental considerations.
- Collaborative Filtering for Geospatial Data: Similar to how recommendation engines work for movies or products, AI-powered collaborative filtering techniques can recommend geospatial datasets, layers, or maps based on user preferences and behavior, enhancing data discovery and exploration.
Conclusion:
The geospatial industry is undergoing a remarkable transformation through the integration of AI technologies such as computer vision, text analytics and NLP, machine learning, and recommendation engines. From enhanced data analysis and interpretation to improved decision-making and personalized recommendations, AI is revolutionizing how geospatial data is utilized. By harnessing the power of these technologies, organizations and professionals in the geospatial field can unlock new insights, drive efficiency, and make informed decisions for a wide range of applications, from urban planning and environmental monitoring to transportation optimization and personalized travel experiences.
Remember, AI is a rapidly evolving field, and as technology progresses, we can expect even more exciting use cases and advancements within the geospatial industry. By staying at the forefront of AI innovations and leveraging its potential, we can unlock the full power of geospatial data and create a smarter and more sustainable future.
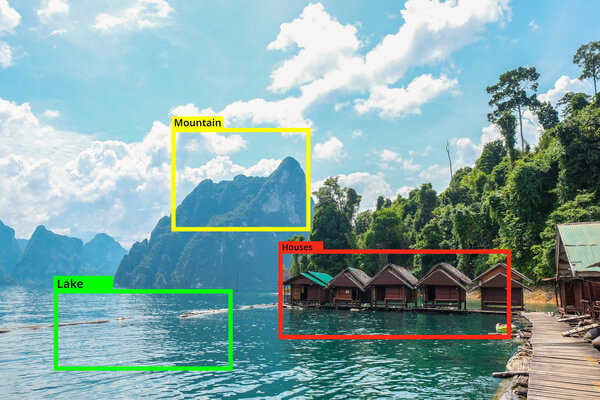
Our commentary
ChatGPT generates 4-5 possible uses of AI across computer vision, NLP and text analytics, machine learning and recommendation engines in the geospatial industry. And just like the last time, they are not very original.
The computer vision examples generated by ChatGPT are common in the industry. We took part in quantifying the impact of mining sites on the environment: water quality, vegetation condition and land cover changes while building the TerraEye platform with one of our clients – FourPoint. What differentiates FourPoint is the usage of data fusion techniques. By combining data from different satellite sensors, their computer vision models achieve greater accuracy than models trained solely on single sensor data.
There is a trade-off there, though – the complexity of data ingestion and inference pipelines grows. By managing the pipelines in a smart way, FourPoint is able to reuse the components in other areas, such as measuring land subsidence with millimetre scale precision by leveraging InSAR processing.
For NLP and text analytics ChatGPT gives some of the more well-known use-cases. NER, sentiment analysis and translation tasks are well known. You can read more about sentiment analysis here: Customer Sentiment Analysis: a way to refine your business strategy.
By chaining those functionalities together, some truly amazing things can be achieved. For example, satellite constellation operators often have an option for their satellites to be tasked to monitor specified areas. They can watch social media feeds and news outlets in order to pre-task the satellites to start image acquisition as soon as a major event is mentioned in the media. This ranges from natural disasters and military operations to large-scale public events. By leveraging NLP in this way, they can acquire the data before anyone even asks for it, which would give them an advantage in the market.
We have experience in building event detection and classification models as well as extracting entities from the text. Read this case study to find out more.
Another use-case mentioned by ChatGPT is Geospatial Query Processing. Using natural language to query the data has been a challenge up until recently. With the revolution that’s happening now thanks to the Large Language Models (LLMs) such as OpenAI’s ChatGPT, GPT-4 and Google’s BARD it has now become possible to greatly enhance the user experience by providing actual, well formatted answers to user questions – something that Microsoft’s Bing browser integration with GPT-4 is now providing. By indexing company documents and synthetising the answer using LLMs. companies can provide a much nicer experience for their users. We’ve recently expanded our Text Analytics and NLP offering to include Generative AI.
The ML use-cases are more interesting. Traffic congestion estimation is something that Google Maps and TomTom Navigation systems are offering. Using billions of geo-located data points every day they require both very fast and very accurate models. Temporal Graph Neural networks are currently state-of-the-art here. Spatial clustering and image classification is something we actively do with our partners and clients.
The recommendation engines section generated by ChatGPT presents valid although not too original use cases. Personalised Travel Planning sounds similar to the GetOutside application, that we helped develop for Ordnance Survey. You can read more about it and other applications in OS’s portfolio here: Ordnance Survey: the new era of mapping.
After reviewing the generated use cases we find them to be valid but not too disruptive. Let’s prompt ChatGPT for some out-of-the-box ideas next.
Out-of-the-box ideas
Here are some more non-typical and out-of-the-box AI use cases in the geospatial industry:
AI-Driven Wildlife Conservation:
AI algorithms can analyze satellite imagery and sensor data to track and monitor endangered species, helping conservationists identify habitat corridors, migration patterns, and potential threats to wildlife populations. By leveraging AI, geospatial data, and deep learning models, researchers can gain insights into animal behavior, population dynamics, and ecological changes, contributing to more effective conservation efforts.
Urban Sound Analysis:
AI-powered audio recognition can process urban soundscapes captured by sensors or acoustic monitoring devices. By analyzing the sounds of the city, such as traffic noise, construction activity, or emergency sirens, AI algorithms can provide valuable information for urban planning, noise pollution management, and public safety optimization. This unconventional use of AI enables the creation of more livable and sustainable cities.
Natural Disaster Response and Recovery:
AI can play a crucial role in disaster response and recovery efforts. By combining satellite imagery, social media data, and machine learning algorithms, AI can automatically analyze and prioritize areas affected by natural disasters, such as earthquakes, floods, or wildfires. This information can assist emergency responders in deploying resources, assessing damages, and coordinating relief efforts efficiently.
Agricultural Disease Detection:
AI-powered analysis of satellite imagery and hyperspectral data can help identify and monitor crop diseases in agricultural fields. By analyzing subtle changes in vegetation reflectance patterns, machine learning models can detect early signs of diseases, nutrient deficiencies, or pest infestations. This enables farmers to take proactive measures, such as targeted spraying or precision farming, minimizing crop losses and optimizing agricultural practices.
Geospatial Emotion Analysis:
By combining geospatial data with facial recognition and sentiment analysis, AI can detect and analyze emotions expressed by individuals in specific locations. This innovative approach can provide insights into public sentiment towards events, tourist experiences, or urban environments. Government entities, tourism boards, and businesses can utilize this data to enhance visitor experiences, design urban spaces, or evaluate the impact of cultural events.
Marine Ecosystem Monitoring:
AI and underwater robotics can revolutionize marine ecosystem monitoring. Autonomous underwater vehicles equipped with computer vision and machine learning algorithms can analyze underwater imagery to identify and track marine species, monitor coral reef health, or detect illegal fishing activities. This technology enables researchers and conservationists to gather valuable data from remote and inaccessible marine environments.
Historical Reconstruction:
Using historical maps, photographs, and archival data, AI algorithms can reconstruct and visualize historical landscapes, cityscapes, and archaeological sites. This allows researchers, historians, and urban planners to virtually explore and analyze past environments, understand urban development patterns, and preserve cultural heritage.
Conclusion:
As AI technology continues to advance, the geospatial industry can explore and embrace unconventional and non-typical use cases. From wildlife conservation and urban sound analysis to agricultural disease detection and marine ecosystem monitoring, AI has the potential to unlock new insights, drive innovation, and address complex challenges in the geospatial domain. By thinking outside the box and leveraging AI’s capabilities, we can harness the power of geospatial data to create a more sustainable, efficient, and informed future.

Our commentary
The out-of-the-box ideas are a bit unusual and some of them might be unpractical or impossible to implement in the near future. The Geospatial Emotion Analysis is an interesting concept, but it yields a question about data privacy and human rights. Applications like that should be developed with responsible AI principles.
Historical Reconstruction is an area of active research. LIDAR technology is commonly used here, but the high costs of LIDAR data acquisitions and data processing challenges might make it hard to quickly scale to the mass market. However, the idea that we could walk into a museum or just launch an app to go out and see how historical cities looked like is really appealing.
The other use cases mentioned by ChatGPT are not so imaginative and revolve around themes, such as smart agriculture, environment monitoring or natural disaster monitoring, which are all topics of active research.
To sum up
As we conclude the second part of our exploration of ChatGPT’s prowess in generating use case ideas for the geospatial industry, it is essential to reflect upon what it means for the domain and the artificial intelligence ecosystem at large.
First and foremost, ChatGPT’s ability to generate use cases that are both pragmatic and innovative, albeit somewhat traditional at times, exemplifies the potential of large language models in understanding and contributing to specialised industries like geospatial technology. The generated ideas were varied, ranging from widely recognised applications in computer vision and NLP to more experimental propositions like Geospatial Emotion Analysis and Historical Reconstruction.
Furthermore, the capacity of ChatGPT to generate these ideas points to the promise that large language models hold as tools for ideation and problem-solving. This potential extends beyond a mere idea generation, as the utility of ChatGPT could also be harnessed in an iterative development process, where it collaborates with human expertise to refine and shape groundbreaking solutions.
It is crucial, though, to approach this potential with measured enthusiasm. Large language models like ChatGPT are tools that can catalyse innovation but are not a replacement for the nuanced understanding and domain expertise that professionals bring to the table. A synergistic approach, where AI collaborates with human ingenuity, is likely to be the most effective path forward.
In conclusion, as we look to the horizon, the integration of AI in the geospatial industry holds boundless possibilities. ChatGPT serves as a reminder that sometimes the catalyst for innovation can come from unexpected quarters. Through collaboration and an open mind, the geospatial industry can harness these AI-driven insights to forge new paths and shape the future of a more connected and insightful world.
Stay tuned for our next article in this series where we shall harness ChatGPT’s capabilities to explore another exciting industry. Until then, if you are interested in learning more about our Geospatial Services Offering don’t hesitate to contact us!
About the author
CONTACT US