Regulation of AI in Healthcare in 2024: EU and FDA approaches
In today’s rapidly evolving healthcare landscape, the integration of Artificial Intelligence (AI) is no longer a futuristic concept; it’s an essential resource that industry leaders need to harness to remain competitive. From precision medicine to predictive diagnosis to drug discovery, AI is revolutionising the practice of medicine and redefining the competitive landscape of the healthcare sector.
But these advances are not without risks. Whether you’re a researcher, investor, or medical practitioner, you need to understand the limits of AI technology and the likely directions it will take under the guidance of regulators, who are scrambling to monitor and control the frenetic pace of innovation in the healthcare field.
In this article, written in cooperation with Darya Lialina, Quality and Regulatory Affairs Manager at Spyrosoft, we’ll take a closer look at how AI is applied in the healthcare industry and what implications the new AI regulations will have for the sector in 2024.
What are the benefits of AI in Healthcare?
Artificial Intelligence offers numerous benefits in healthcare, revolutionising the industry in various ways. Some of them include:
Diagnostics and disease detection
AI can analyse medical images, such as X-rays, MRIs, and CT scans, to assist in the early and accurate detection of diseases like cancer, diabetes, and cardiovascular conditions.
It can provide rapid and consistent image interpretation, reducing the chances of human error and improving diagnosis.
Personalised treatment and medicine
AI can analyse patient data, including medical history, to develop personalised treatment plans and suggest the most effective medications and therapies. Personalised medicine can enhance treatment outcomes and minimise adverse effects.
Predictive analytics
AI can forecast disease outbreaks, patient readmissions, and medical equipment maintenance needs by analysing vast amounts of data. This enables healthcare providers to allocate resources efficiently and improve patient care.
Virtual health assistants and chatbots
AI-powered virtual assistants and chatbots can provide 24/7 support, answer patient queries, and offer health advice. They can help alleviate the burden on healthcare professionals and improve patient engagement.
Remote monitoring
AI enables remote patient monitoring, allowing healthcare providers to keep track of patients’ vital signs and health status in real-time. This is particularly valuable for managing chronic conditions and ensuring early intervention.
Read more about other applications of AI in healthcare the benefits they bring.
Partner with us for AI regulatory compliance
Find out moreUnderstanding the Risks Posed by the Use of AI in Healthcare
The biggest benefit of AI systems, and at the same time their biggest risk, lies in their capability to automatically learn from vast amounts of training data generated during the routine delivery of medical services. This hands-off approach presents a number of risks that are novel and unique to this technology, including:
- Lack of explainability – Due to this purely data-driven approach, it is often unclear what exactly the model learns and how it will behave in an environment that is different from the one in which it has been created. Due to this lack of explainability, modern AI systems are often described as opaque or as a “black box”, whose inner workings remain elusive.
- Bias – First of all, there is a notable lack of diversity in the electronic health record (EHR) used to train many AI/ML enabled healthcare systems, which in turn can result in biased outputs. Additionally, the misuse of certain features present in the data can skew the results of an algorithm, such as when money spent on healthcare was included among factors for estimating the level of illness in patients by one popular algorithm. This led the algorithm to conclude that people of colour, who tend to spend less money on healthcare, were less ill than white patients presenting similar conditions.
- Poor predictive performance – All AI and ML systems have irregular predictive performance due to confounding factors and domain shift. For example, a machine learning (ML) model that was trained to successfully predict hip fracture from radiographs primarily relied on confounding factors such as the CT scanner model or patient age, which the ML model could infer from the scans. When deployed in a hospital with different CT scanners or different patient demographics, a problem known as domain shift, such a model cannot be trusted to make correct predictions.
- Lack of clear regulations – Universally applicable AI guidelines (or even principles) do not exist, much less any that would apply to the healthcare sector in particular. Researchers and industry leaders are often in a position of having to anticipate the future rules that might replace current guidelines or apply to currently unregulated areas. Technical and business decisions taken today may prove fatal if they conflict with future, or unknown rules.
- Competency gaps – Computer scientists who create AI systems typically lack medical knowledge while clinicians usually possess limited understanding of complex algorithms. The business side of a project may lack knowledge in both domains. Building safe and performant AI healthcare tools requires substantial interdisciplinary collaboration and knowledge transfer.
Overall, a general understanding of these risks by all stakeholders is essential in reducing the likelihood of incorrect diagnoses, ineffective treatments, and other undesirable behaviour by AI systems. Additionally, the people building and marketing AI healthcare systems need to have their finger on the pulse of regulatory trends in their jurisdiction.
Fundamentally, regulations should address risks – to health and safety, to the environment, to the economy, to consumers, etc. – and their causes. Rules and procedures that are based on science, focused, and proportionate are more effective, and less costly.
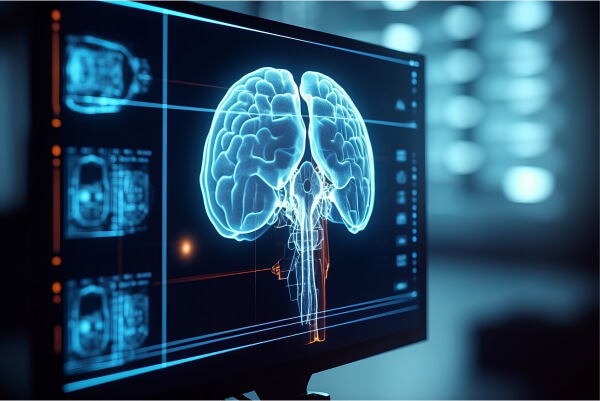
Current and Proposed US Regulations for the Use of AI in healthcare
The United States regulates industry in a particular manner. The individual states are the primary source of legislation on the issues of public safety, health, welfare, but the federal government is charged with regulating interstate and foreign commerce. Owing to this unstable balance of power, overambitious federal legislation is often rejected by the court. In the void left by centralised legislation from the federal government, agencies of the executive branch are able to regulate activities important to the interests of the nation, and in some cases, to pre-empt state laws.
One such agency is the Food and Drug Administration (FDA), which is acutely interested in forming regulations and policies surrounding AI in medical applications. The FDA has approved 692 AI/ML-enabled medical devices (as of October 2023), with more than 80% of them since 2019 (marketed via 510(k) clearance, granted De Novo request, or premarket approval). It is worth noting, however, that as of this writing, the FDA has not approved any devices that rely on a purely generative AI architecture.
The list of AI/ML-enabled medical devices marketed in the United States can be found here.
In its 2021 action plan, the FDA outlined five specific goals for the practical oversight of AI/ML-based Software as a medical device (SaMD)
- Tailored Regulatory Framework – The proposed regulations focus on the problem of AI systems that learn over time and require a “Predetermined Change Control Plan”, which has recently been published as a draft guidance. This plan needs to outline “what” aspects of the AI system can be adapted through learning and has to explain “how” exactly the system implements adaptation while remaining safe and effective
- Good Machine Learning Practices – Ten guiding principles are proposed that promote safe, effective, and high-quality medical devices. One of these principles is that “Clinical Study Participants and Data Sets Are Representative of the Intended Patient Population”, which addresses the aforementioned domain shift problem. Another principle states that “Focus Is Placed on the Performance of the Human-AI Team” and promotes human interpretability of the system and therefore encourages the development of transparent rather than opaque black box ML models.
- Patient-Centered Approach With Increased Transparency – Transparency of AI systems is crucial not only for clinicians but for patients as well. Just as patients can ask their doctor why they, for example, recommend a certain treatment plan they should be able to ask that question to an AI system that made that recommendation. This is sometimes referred to as a “right to explanation”, similar to the one found in the EU’s GDPR, as mentioned below.
- Reducing Algorithm Bias – AI/ML systems, which are usually trained with data from historical datasets, can be susceptible to biases. This is because they can reflect the biases that are inherent in the data they are trained with. Given that healthcare delivery is often influenced by aspects such as race, ethnicity, and socio-economic status, there are chances that the present biases in our healthcare system could unintentionally be integrated into these algorithms.
- Real-World Performance – Collecting real-world performance information regarding the practical application of SaMD can provide manufacturers with insight into their products usage, highlight areas for enhancements, and help them act promptly on safety or user-friendliness issues. This is again related to the domain shift problem, since a model’s performance that is satisfactory in the training environment may degrade severely when the model encounters unseen data.
State-level AI regulations
Turning briefly to legislative efforts at the state level, a notable example comes from
AB 311, concerning “Automated decision tools,” currently under consideration by the California Assembly. The law would apply to both public and private deployers of automated systems, in the healthcare sector among others, and would require them to:
- Submit annual impact assessments
- Communicate the reasons behind each of the system’s decisions, if the subject of the decision is a natural person
- Allow the subject of the decision the opportunity to be considered by a non-automated system, if they are a natural person
- Develop safeguards, document the system’s limits, and communicate them to users and subjects of the system
- Establish a designated governance / compliance person
- Draft a publicly available policy describing the tools used, their risks, and the steps taken to mitigate those risks
The Executive Order on safe, secure, and trustworthy development of AI
One of the most recent actions aimed at regulating AI in the healthcare sector in the US has been issuing the Executive Order on safe, secure, and trustworthy development of Artificial Intelligence by President Joe Biden. The order outlines several key actions to achieve this goal. Below you can find a summary of the most important ones from the perspective of the healthcare industry:
New Standards for AI Safety and Security:
- The requirement for sharing safety test results and critical information with the government ensures that AI systems used in healthcare are safe and trustworthy.
- Development of standards, tools, and tests for AI safety and security is particularly relevant for healthcare, where patient safety is paramount.
Protecting Americans’ Privacy:
- Prioritising the development of privacy-preserving techniques in AI can help safeguard sensitive patient health data.
- Strengthening privacy-preserving research and technologies is vital for protecting patients’ medical information.
Advancing Equity and Civil Rights:
- Healthcare AI applications must address issues related to discrimination and bias, especially in areas like diagnosis and treatment recommendations.
- Ensuring fairness in the use of AI in healthcare is critical to maintaining equitable access and outcomes for all patients.
Standing Up for Consumers, Patients, and Students:
- The responsible use of AI in healthcare can lead to the development of life-saving drugs and innovative treatments.
- AI-enabled educational tools can help train healthcare professionals and improve healthcare education.
Promoting Innovation and Competition:
- Principles and best practices for AI should include considerations for healthcare workers, as AI is increasingly integrated into medical practice and administration.
- Addressing job displacement in healthcare is essential to support workers during the adoption of AI technologies.
Ensuring Responsible and Effective Government Use of AI:
- Government guidance for AI use can influence how federal agencies and healthcare organisations employ AI technologies.
- The acquisition of AI products and services is pertinent for healthcare agencies looking to adopt AI solutions.
The most critical elements of the Executive Order for healthcare are those that ensure the safety, security, and ethical use of AI, as well as those that promote equitable access and outcomes for all patients.
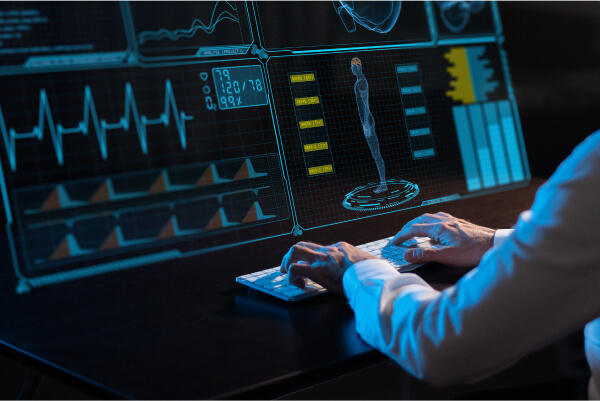
How the EU Will Regulate the Use of AI in the Healthcare Field
The European Commission, the European Medicines Agency (EMA), and the International Coalition of Medicines Regulatory Authorities (ICMRA) are working together to develop coherent policies and regulations addressing the complex challenges of AI use in healthcare. Some of the current suggestions for future regulations include:
- Enhanced oversight – AI healthcare businesses should have a multi-disciplinary oversight committee to oversee product development to understand and manage the implications of higher-risk AI. Regulators will establish guidelines for the designation of a Qualified Person to oversee AI / ML compliance
- Explainability – The performance of so-called “black-box” models may justify their use in some cases, but wherever possible, developers should make an effort to unveil the inner-workings of their tools. Some recommendations include the provision of feature importance lists, SHAP and/or LIME analyses, and similar explainability metrics, not just for the model but also for individual inferences during deployment.
- Data Integrity – Future rules on data provenance, diversity, authentication, validation, protection, and usage should follow the principles of necessity (data should be strictly relevant), proportionality (collect only the data that’s needed) and subsidiarity (use the least sensitive data possible).
- Human in the Loop – The inclusion of human agency and oversight within the development and deployment of AI/ML tools will help to build trust in their effectiveness, reliability, and fairness. This “human-centric” approach should extend beyond collection and modelling to also encompass a reliance on user and patient reported outcomes in the evaluation of AI/ML tools.
- International Standards – Regulators should encourage the international development and standardisation of sound machine learning practices in the healthcare industry under the principles of reliability, quality, transparency and understandability. Medical device classification rules should depend on factors such as human oversight, safeguards, self-learning, and autonomy.
Many of these proposed guidelines are currently being considered in the proposed AI Act by the European Commission. The draft of the AI Act defines as concrete objectives 1) to create a legal definition of “AI system”, where a working definitions is a …software that is developed with [specific] techniques and approaches and can, for a given set of human-defined objectives, generate outputs such as content, predictions, recommendations, or decisions influencing the environments they interact with. And 2) to adopt a risk-based approach that proposes different regulations depending on the following risk categories:
- Unacceptable risk – AI is harmfully manipulative, exploits vulnerable groups, implements social scoring for public authorities or uses biometric identification systems for law enforcement)
- High risk – AI used as safety component or in certain areas such as categorisation of natural persons or management of critical infrastructure
- Limited risk – Chatbots, Generative AI for image audio or video
- Low risk – Limited or peripheral use of AI or ML
AI systems with an unacceptable risk will be banned as they are considered a clear threat to people’s safety. Providers of high-risk AI systems will be required to register in a EU-wide database and such systems are subject to a range of requirements particularly on risk management, testing, technical robustness, data training and data governance, transparency, human oversight, and cybersecurity. Applications associated with a limited risk are only subject to a limited set of transparency obligations, whereas low risk ones are free from any obligations.
Finally, the EU General Data Protection Regulation (GDPR) guarantees the right to “meaningful information about the logic” of algorithmic systems. This can be interpreted as “a right to explanation” where automated decisions by AI must be accompanied by a human-understandable explanation. Furthermore, GDPR states that algorithmic systems must not make significant decisions that affect legal rights unless there is some level of human supervision.
Partner with us to ensure that your software is complaint with AI regulations
As shown above, the guiding principles behind the regulations being formulated by the US and the EU are similar. While the direction of regulations in the EU is relatively clear, it is too early to tell which principles will be formally implemented into US law, and when. As of this writing, most of these principles only exist within statements of policy, and are not enacted. The most notable exception is the EU’s privacy law (GDPR) which already includes requirements relating to the explainability of AI systems and the need for human involvement in some automated decisions.
Rapidly evolving AI technologies represent unique opportunities for novel software solutions for healthcare analytics, personalised medicine, decision support systems, and other uses of AI in medicine. At the same time, software developers and computer scientists must be cognizant of incoming regulations surrounding AI in the healthcare industry. Spyrosoft has a longstanding track record of meeting this two-pronged challenge, and is the ideal collaborator for businesses seeking to leverage AI in the healthcare sector.
Our experience in creating software solutions for medical and in vitro diagnostic medical devices (IVD) demonstrates a nuanced understanding of the complex needs of this industry. Along with our expertise in software development, we provide comprehensive services from Quality Management System (QMS) to regulatory support. Partner with us to pave the way forward in AI healthcare.
About the author
Contact us