AI and machine learning in healthcare: transforming patient care and revolutionising medical research
AI and machine learning are bringing novel solutions and remarkable progress to the healthcare industry – from early diagnosis and disease prevention to personalised treatments and disease development prediction. By analysing large datasets, AI can discover complex patterns and learn from them, providing insights to healthcare professionals who can make more informed decisions and reduce medical errors.
In this article, prepared in cooperation with Tomasz Smolarczyk, Head of AI and ML at Spyrosoft, we’ll explore various applications of AI in healthcare and discuss the benefits it brings.
Let’s dive in.
Computer vision
Medical imaging
AI technology can be used to analyse images, for example, of skin lesions, and classify it as a cancerous or non-serious change. Patients can upload images to a secure platform, where AI can triage and prioritise patient cases and help doctors diagnose patients remotely. It may significantly cut down the time and reduce the need for follow-up tests.
Another example of using AI in medical imaging is a solution that analyses microscopic images of a blood sample and counts the blood cells, helping diagnose diseases early and accurately. It could be used to diagnose malaria – a life-threatening disease caused by a parasite transmitted through the bites of infected mosquitos. Early diagnosis is critical for preventing severe damage to health or even death. Thanks to AI-powered solutions, the diagnosis can be made faster and more accurately, particularly in areas where access to medical staff is limited.
AI can be used to analyse X-ray images or other medical scans to detect, for example, the first signs of cancer. AI can also automate doctors’ work on annotating medical images, marking up areas with visible changes that will be treated with radiation and healthy areas that may get damaged. With models such as Segment Anything Model (SAM) developed by Facebook, we can expect an increasing number of AI solutions that will speed up the labelling work needed to be done by medical experts.
In dentistry, AI-powered medical imaging can potentially make treatment more personalised, thus, more accurate. For instance, it can help match implants to individual patients’ teeth by scanning their mouths. It can also be used to create a 3D model of a patient’s mouth, giving a better insight into a patient’s oral health – AI can detect cavities, gum disease, and other issues.
Movement analytics
Some time ago, our team was working on a project called MedBand – a wearable device used to track patients’ movements when doing rehabilitation exercises and compare it to the model way of doing the rehabilitation training. The solution helps patients exercise correctly and effectively and reduces the risk of injury due to incorrect movements.
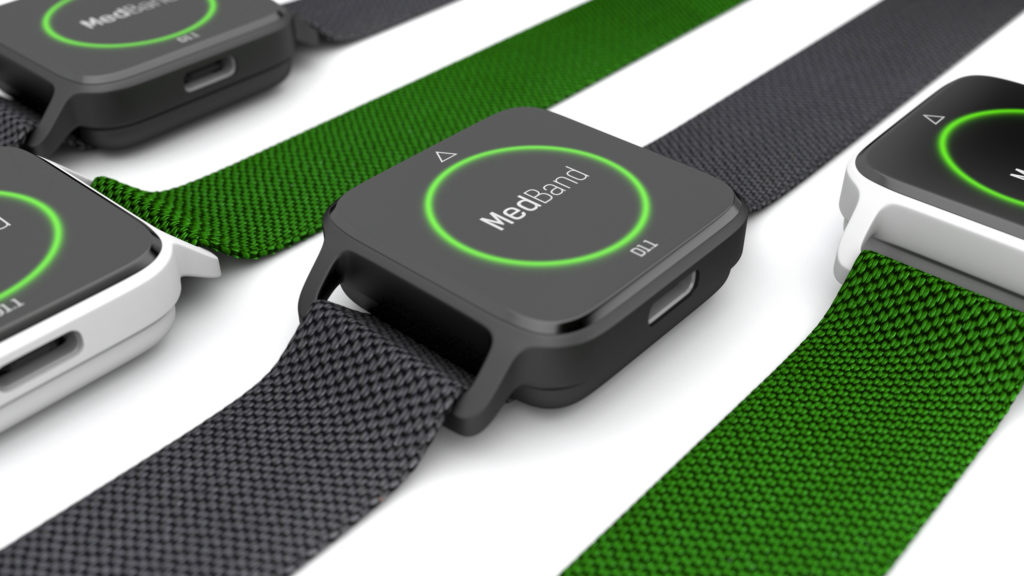
Sports analytics
AI can be used to monitor athlete’s fatigue levels by analysing their performance and biometrics, which may help identify potential injury risks. Based on these insights, trainers can adjust the training plan or identify at-risk players and take steps to reduce the injury risk.
Discover how we used computer vision for badminton instant review system. >>
Leverage the power of AI in your solution
Get more detailsText analysis and natural language processing
Information extraction
AI can extract key information, which refers to disease symptoms or illness, from a piece of text or a voice recording. It may be especially useful in telemedicine, when during a call with a patient, a doctor can access the information extracted from a patient’s medical history. Also, the symptoms that a patient mentions during the online medical interview can be extracted from the conversation and automatically assigned with a respective clinical code. We all know that doctors’ time is precious and with the AI acting as their co-pilot taking notes, doctors can devote more time to their patients rather than note-taking.
Recent progress in developing large language models has demonstrated that AI can successfully retrieve medical knowledge and provide accurate answers to medical questions backed by reasoning. Recently, Google has published a second iteration of their LLM model called Med-PaLM 2, which is based on a similar model as the trending ChatGPT. Its predecessor, Med-PaLM, was the first to obtain a “passing score” (>60%) on U.S. medical licensing-style multiple-choice and open-ended questions. Recently, Med-PaLM 2, consistently performed at an “expert” doctor level on medical exam questions, scoring 85%.
Recently, Google has also presented a demo of a new solution for step-by-step orchestration of search and conversational applications with pre-built workflows. One of its core functionalities is summarising the essence of reports and other multi-page documents into a few concise sentences. In the healthcare industry, this could be used to summarise scientific papers published in the PubMed database, which could then be suggested to doctors as recommended readings when they are treating patients with rare or unusual medical conditions. The solution could also be used to condense patient’s long-term medical history or medical documentation into a summary of key facts. Again, that would be a huge time saver for the medical staff.
Text classification
AI can help classify medical data into categories, for example, patient description, treatment description and symptoms observed throughout the patient’s medical history. It can also be applied to other related areas, like medical invoice processing and classification according to specific categories, for easier management.
Clinical decision support
AI has a great potential to support doctors not only at the diagnosis stage, but also throughout the treatment. It can analyse the electronic health records (EHR) to create personalised treatment plans for patients based on their medical history, lab results and other relevant data, for example, available records of patients with the same disease who recovered.
Sentiment analysis
AI can help analyse patient sentiment from a video recording or text. During a videoconference with a patient, AI can detect what emotions the patient feels by analysing their facial expression, for example, whether they are confused, and a doctor needs to repeat or paraphrase the question.
AI-powered sentiment analysis can be used in mental health apps. By monitoring the sentiment of a user’s responses during a chat with a doctor or a bot, it can be assessed if their mental health is deteriorating and adjust the therapy accordingly.
I firmly believe that integrating AI as “copilots” in healthcare processes can significantly improve the patient journey and enable medical professionals to provide high-quality care while simultaneously easing their workload.
Despite the continued necessity for human touch in healthcare, those who embrace and leverage AI capabilities will emerge as industry leaders and drive progress towards a more efficient, effective, and patient-centred healthcare ecosystem.
Tomasz Smolarczyk, Head of AI at Spyrosoft
Machine learning and applied data science
Classification
By analysing data, AI can filter out certain patterns, based on which it may make predictions about similar cases. For example, we can create a model that would analyse data about patients who died of cancer and use the identified patterns to prognose disease development in patients with similar characteristics. With these insights, doctors can make decisions about changing or adjusting the therapy.
Prediction models can also be used to prognose how long certain disease symptoms will last or when a patient will be able to leave the hospital. Another use case for the predictive models is readmission rate monitoring. Patient readmission to a hospital might be a characteristic of a given condition or may be a result of incorrect performance of a medical procedure and may indicate poor quality of services, so for medical institutions it’s key to keep it under control.
Predictive maintenance
Modern medical equipment is very expensive, and any technical defect may be a threat to patients’ health and life, that’s why the prevention and early detection of failures is critical.
The use of AI for predictive maintenance in healthcare can help reduce equipment downtime, minimise unexpected equipment failures, and ensure that medical devices are always in good working condition. This can lead to better patient outcomes, as well as cost savings for healthcare facilities.
An example of predictive maintenance in healthcare is the use of sensors to monitor the performance of medical devices. The sensors detect unusual behaviour, like vibrations or temperature rise or other factors that may indicate potential issues with a device. The collected data can be then analysed by the machine learning algorithms to predict when maintenance or repair is needed.
Sales forecasting
Sales forecasting is especially important in the pharmaceutical industry due to the high costs involved in drug development and the long process of bringing a new product to the market.
Since AI can analyse large amounts of data quickly and accurately, it allows companies to make more informed decisions about product strategy. AI can generate forecasts of future sales, taking into account historical data and market trends.
Simulation
With AI, we can simulate certain situations to see various scenarios. It allows us to generate multiple solutions, which we can compare and choose the best one.
An example is the solution we worked on together with the University of Life Sciences in Wroclaw. We combined the data on the mobility of people with the SIR model for the spread of an infectious disease. By knowing when, where and how long people spend time in a specific place, what the contagion risk is, how long the disease lasts, etc. we could use AI to simulate various scenarios of epidemic development. The model not only helps to identify steps that can be taken to limit the spread of a disease, but also to predict the economic consequences of a lockdown.
Recommendation engines
Personalised medical therapy
Having a complete view of the whole patient (other diseases they have, their treatment history so far, other drugs they’re taking), a doctor could adjust the treatment to a specific patient case. One of the other possible applications of AI in personalised medical therapy is adjusting the drug dose to an individual patient, taking into consideration their weight, age, etc.
AI can also be used to analyse data collected by a wearable device, such as a smartwatch, to detect trends or patterns and alert patients or doctors about potential health risks.
Drug discovery and development
Recommendation engines can analyse a database of patients to identify potential candidates for new drug testing based on their genetic data, clinical trials, etc.
Moreover, AI can drive and accelerate new scientific discoveries, as the recent AlphaFold case proved. The project goal was to create a solution – the AlphaFold system – to predict the 3D structure of a protein based solely on its genetic sequence. It uses large genomic datasets to predict protein structure and it creates very accurate 3D models of those proteins. It’s a ground-breaking achievement as it allows us to better understand the protein’s shape and role within the cell, and, thus, develop drugs that will work with the protein’s unique shape and be more effective.
Augmented reality
Medical training
Augmented reality powered by AI can be used to simulate medical procedures, so medical students can practice surgical techniques in a realistic but low-risk environment. The simulation can provide feedback on technique, timing and accuracy. It’s likely that AR will become a popular and important tool in medical education soon.
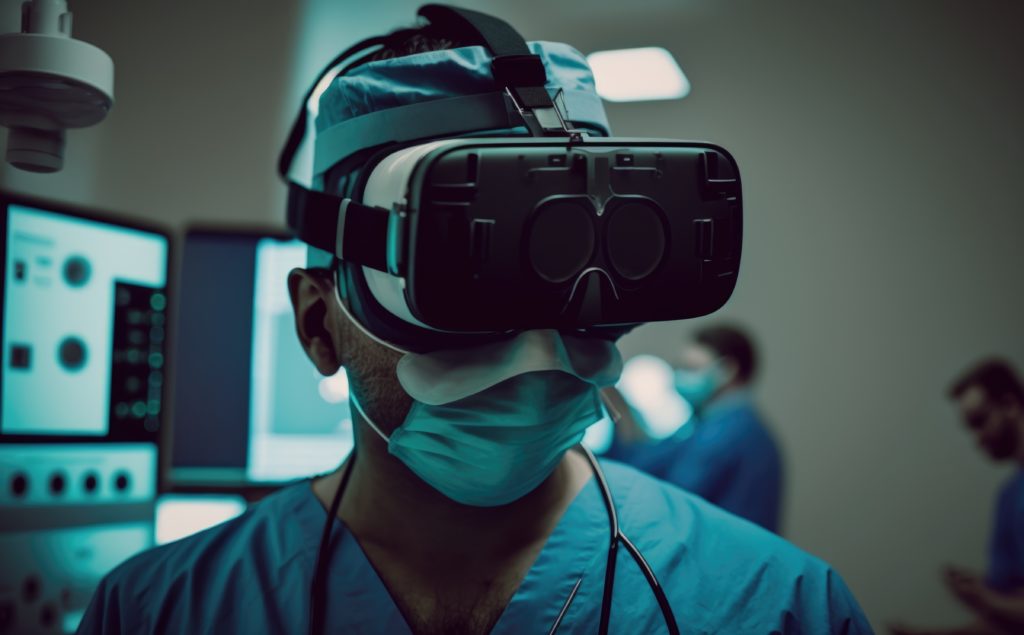
Leverage the power of AI in healthcare
Our experts will advise you on how to best apply AI in your specific business case. Contact us via the form below.
About the author
Contact us