Customer Lifetime Value prediction: the key to higher profits and growth
Analysis, computation and Customer Lifetime Value prediction are essential facets of the business planning methodology of all financial institutions, including fintech companies. All fintech companies are required to invest time, money and effort in customer relationships to garner revenue and ensure the profitability of the entire enterprise.
What is a Customer Lifetime Value?
Customer Lifetime Value (CLV) refers to the approximate profit that an organisation can derive from a customer over a well-defined period of time. The term ‘lifetime’ indicates that this value is calculated for all transactions throughout the relationship with a customer. However, in practice, CLV is computed for a period of anywhere between 1-5 years, depending on the requirements and plans of different organisations.
The customer acquisition plans and strategies of fintech companies must take into account the CLV attributed to different customers as well as groups. An accurate prediction of CLV has a tremendous role because it helps fintech companies segment customers using the quantified values that a specific group of customers is expected to add to company revenue.
Why you should know a CLV for your customer segments?
CLV prediction also plays a vital role when it comes to selecting and promoting relevant services to different customer segments. When a financial institution is able to quantify the total expected profit that a customer can bring within a specific period of time, they can plan the expenses, and the time and effort that can be put in, proportional to the expected profits.
The marketing strategies of fintech companies can also become more effective when they factor in CLV because they will become aware of the expected profits. As a result, they can allocate marketing budget accordingly because there is no guesswork involved in preparing it.
Fintech companies can drive up their revenues and profits by using predicted CLVs. They can identify the most profitable customers and invest in acquiring and retaining them.
CLV is useful for the present as well as future business strategies of fintech companies. Customers who are highly profitable now may not be at the same level in the future. Similarly, customers who aren’t contributing significant revenues now might become extremely profitable over time because of their expected career growth.
With high-quality and accurate analysis and prediction of CLVs, fintech companies can find the key to unlocking continued and consistent profits.
How to calculate your Customer Lifetime Value?
On the surface, calculating and computing CLV seems to be a simple task. There are many methods available for calculating these figures. I’ll go through the most popular one below.
Customer Lifetime Value can be calculated when you multiply the average customer lifespan by customer value.
Before, you’ll get to estimating this number, let’s go through all the metrics you’ll need.
Average customer lifespan
To calculate this number, you need to divide the sum of customer lifespans by the number of customers.
Average purchase frequency rate
This one is also easy to estimate – to do that, divide the number of purchases by the number of customers.
Average purchase value
Average purchase value can be calculated by dividing your total revenue by the number of orders.
Customer value
And yes, you can finally work out your customer value that will allow your customer lifetime value. To determine this number, you’ll need to divide the average purchase value by your average purchase frequency rate.
There it is, you’re now ready to calculate the customer lifetime value. To do that, divide the average customer lifespan by the customer value.
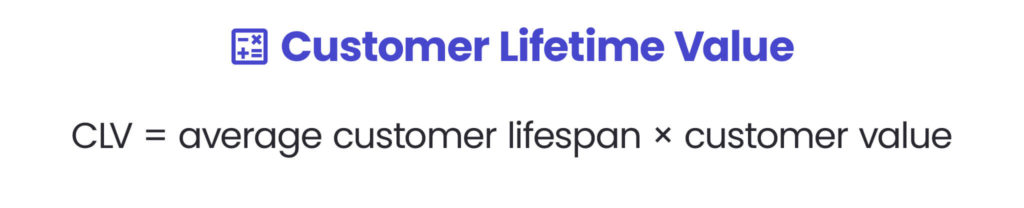
Even this method is quite accurate in itself, the final result isn’t as relevant as it could be because quite often the accuracy of variables in these methods is questionable. At the same time, accurate analysis of CLV and formulating strategies after it’s established, is often a complicated task.
They also don’t get us any closer to the real deal in business: Customer Lifetime Value prediction.
What if you could be able to not only calculate current CLV for your customer value, but also predict it for existing and future customers from different segments?
This is where the process gets tricky.
Problems faced by fintech companies in Customer Lifetime Value prediction
Even though some of these methods are accurate in themselves, their results aren’t as relevant as they could be because quite often the accuracy of variables in these methods is questionable. At the same time, accurate analysis of CLV and formulating strategies after it’s established, is often a complicated task.
At this juncture, Big Data analytics and Machine Learning tools can be brought into the picture to make CLV calculation accurate and beneficial to the business.
Some of the major drawbacks leading to inaccurate computation or usage of CLVs are listed below:
Inaccurate data
Depending on the method of calculation to be used, different variables about customers and customer groups need to be utilised. Average Order Value (AOV), Purchase Frequency, Churn Rate, Customer Lifetime, Repeat Rate and Customer Loyalty among others, are the most frequently used metrics in the computation of CLVs.
If the numeric value of any of these metrics isn’t accurate, the results will never be correct. So, the use of data sciences in general and Big Data analytics, in particular, can eliminate this drawback.
Improper segregation
After the calculation of CLVs, fintech companies need to focus on segmenting customers and groups based on profitability. It is vital that present, as well as future profitability, is taken into account for formulating strategies and defining the criteria for segmentation. Many financial institutions fail to get this done accurately.
Estimating the ideal time period for CLV calculation
When CLV is calculated for long periods, it may not show the true picture. The same is true in the case of CLVs calculated for extremely short periods.
Depending on the nature of business and the services to be offered, the time for which CLV is calculated needs to vary. FinTech companies need to focus on this aspect to a great extent if they want to enjoy all the planning benefits that they can get from the CLVs.
Planning and usage of CLV
Even if you have accurate data and the CLV calculated is viable, implementing and incorporating it in practical business plans is essential. FinTech companies need to make use of the available information in the most viable and profitable fashion to garner optimum benefits.
How Big Data analytics can be helpful?
Big Data analytics and Machine Learning tools have gradually become an integral part of computing and predictive Lifetime Values for groups, organisations and individuals.
With the use of these methods, fintech companies can rest assured about the accuracy of all metrics used in the analysis and calculation of CLV. They can also shed light on the ideal categories for the segmentation of different customer groups. As a result, fintech companies can optimise the services they offer to these groups and segment the most profitable customers with ease.
Establishing the ideal time period for estimating CLV makes a significant difference to the eventual outcome. The use of Big Data analytics can also ensure that financial institutions and fintech companies make use of the most viable and relevant time period at all times.
Notably, when CLV is calculated for different purposes the optimum time period may vary. For instance, if you want to use this data for marketing purposes and customer acquisition, a shorter time period is sufficient. For customer retention and optimising services, calculating CLVs over a longer period is more effective.
Big Data analytics will help leverage the right methodology depending on the objectives of your organisation.
How does Spyrosoft approach Customer Lifetime Value?
Here’s how our Head of AI, Tomasz Smolarczyk explains it:
Spyrosoft’s expertise in Big Data analytics and Machine Learning tools makes us an asset to all fintech companies looking for optimised predictions of Customer Lifetime Values.
As your service providers, we can guarantee the optimum level of accuracy in all the datasets used to determine the value of different metrics used in CLV calculation. Our team of professionals have proven their value when designing tools to process large volumes of data and in providing the highest standards of Big Data analytics services.
At Spyrosoft, we understand and acknowledge that different organisations require different methods for optimum analysis and computation of CLV.To prepare viable business strategies for different areas, slight adjustments are needed in the evaluation process of CLV, and we are always up to this challenge.
About the author
RECOMMENDED ARTICLES