AI in product development: how gen AI is revolutionising the process
Generative AI and AI tools are reshaping how businesses approach product development, shifting the focus from manual iteration to data-driven decision-making.
In this article, I will explore what this means for companies looking to integrate AI into their development pipelines. I will draw on the speakers’ expertise from the AI Connect conference: Piotr Tański, Principal HMI Software Engineer at Spyrosoft and Peter Schneider, representing our strategic partner, Qt Group. Discover their insights on the future of graphical interface development.
How gen AI transforms the product creation process
Gen AI is changing the rules of the game in product development. It accelerates critical stages such as UI design, code generation, and software testing. Tasks that once took weeks can now be completed within minutes. As Peter Schneider from Qt highlighted during his presentation, today gen AI enables, among other things, the creation of web and mobile applications using natural language.
However, its impact goes beyond that. AI is reshaping the entire product development lifecycle – from initial concept to implementation and updates. Automating key processes, such as code analysis, test generation, and debugging, enhances development efficiency. This translates not only into faster time to market but also into improved quality.
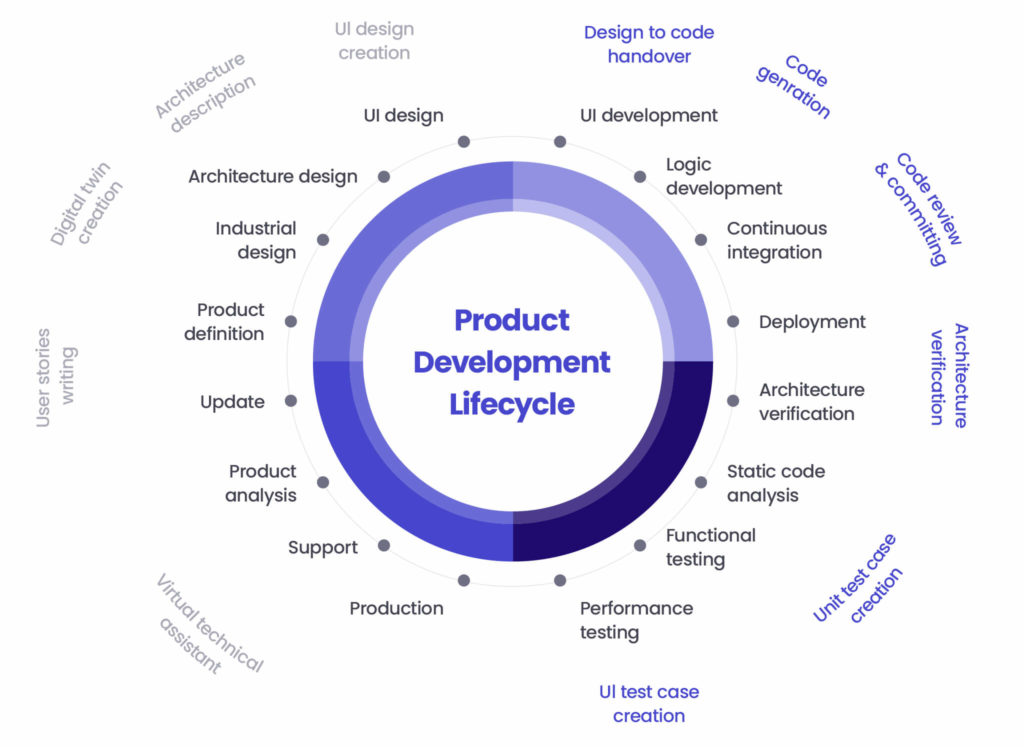
For technology leaders in product-based companies, this should serve as a clear signal: adopting gen AI is becoming essential for maintaining market competitiveness. In their position, I would focus on the following key benefits:
- Speeding up UI prototyping – gen AI enables the automatic generation of UI prototypes based on textual descriptions, significantly accelerating design iterations. Businesses can test concepts more quickly and refine products to align with user expectations, saving both time and resources.
- Faster testing and improved accuracy – AI-powered testing tools can automate repetitive tasks and generate thousands of cases in minutes, reducing manual effort and the risk of post-launch issues.
- Transforming product lifecycle management – AI does not merely generate UI elements; it also analyses user interactions and provides real-time optimisation suggestions. This allows businesses to dynamically adjust interfaces based on evolving customer expectations, moving away from static product roadmaps.
I believe that AI in product development is no longer just a tool for automation – it represents a fundamental shift in how companies design and manage user interfaces.
Challenges in AI implementation
Despite the benefits I have presented, companies still hesitate to use generative machine learning in product development. Although up to 65% of professionals report using gen AI in their work, only 13% apply it specifically in software engineering. During their presentation, our speakers explored the reasons behind this trend, identifying three key factors:
- Gen AI models lack awareness of specific products and have no inherent knowledge of them.
- Product creators are hesitant to input their data into AI models due to concerns about data leakage, loss of competitive advantage, or intellectual property risks.
- Many companies are not yet culturally or organisationally prepared for gen AI adoption.
Despite these challenges, large language models have the potential to significantly improve the analysis and decision-making processes in product development by processing extensive product data and market information.
I should also add that popular LLMs successfully handle only 30–50% of tasks requiring programming expertise. It raises the question of how these models can be integrated into UI/UX development workflows.
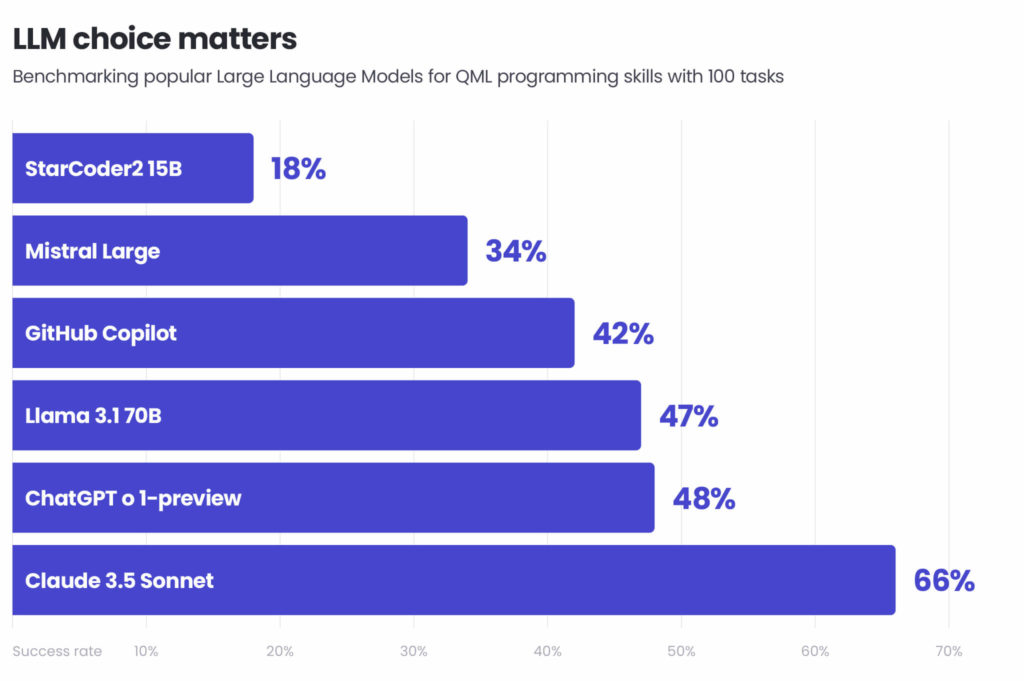
Prompt engineering and fine-tuning: enhancing AI’s effectiveness
I see the opportunity to meet these challenges in advanced prompt engineering and fine-tuning. As our experts highlighted during the presentation, these methods work together to improve AI’s accuracy and usefulness – whether in code generation, data analysis, or process automation. While prompt engineering refines input instructions to guide AI responses more effectively, fine-tuning enhances contextual understanding and accelerates the implementation of new solutions, ultimately leading to more precise and efficient results.
These methods are crucial for optimising various phases of product development, from ideation to market launch.
As a leader in HMI solutions, Spyrosoft demonstrates that combining these two techniques not only optimises daily workflows but also enables the development of innovative products tailored to specific business challenges.
This is due in part to our collaboration with the Qt Group, with whom we are engaged in numerous joint projects. We actively support each other’s efforts in advancing Qt technology, sharing business opportunities, and managing workloads related to customer inquiries. Our partnership is focused on boosting mutual commercial benefits, optimising technology use, and delivering enhanced services to the Qt user community.
Conclusion
Technology leaders should prioritise integrating gen AI throughout key stages of the product lifecycle. Automating UI design through AI-generated prototypes significantly shortens development cycles and reduces costs. Additionally, gen AI can improve prototyping and development, and reduce time needed for quality assurance. This is why, at Spyrosoft, we see this technology not merely as a tool for automation but as a strategic enabler for optimising product management workflows.
About the author
CONTACT US