AI in finance: how can AI improve modelling and forecasting in financial services?
AI has great potential to revolutionise the financial industry. It helps companies cut down operational costs and drive revenue. Thanks to AI-powered solutions, financial companies can automate certain processes and get deeper data-driven insights, which can be used for making accurate predictions and forecasts about future outcomes.
AI can help firms from the financial sector to generate more income, optimise their current processes and cut down certain operational costs. It can be applied across the whole company – from the front office to the back office.
Let’s take a look at some of the top AI use case examples from the financial industry sector.
Fraud detection
In the financial services sector, AI predictive models are often used for fraud detection. They can analyse data in real time to detect suspicious transactions or spot any fraudulent activity. The machine learning model can recognise certain inconsistencies in the usual transactions, for example, that a transaction is made from an unusual location or for a suspicious product.
Churn prediction
AI can use machine learning algorithms to create predictive models that identify the factors that are most likely to lead to customer churn, such as customer demographics, account activity and customer service interactions. Also, by analysing large amounts of data, from example the transaction history, AI can help identify customers who may churn.
Based on the analysed data AI can help financial companies offer customised products and services that meet the preferences of specific customer segments. It can also notify account managers when a customer is showing signs of churn, so they can take proactive steps to retain them before they leave.
AI in finance: creditworthiness assessment
AI predictive models can also help financial companies evaluate their clients’ creditworthiness. The idea is to predict the likelihood that the debtor will repay the money on time, so the creditor can assess how much money they can lend. To determine the credit score, the AI algorithms take into account: the borrower’s credit and payment history, their income and employment status, debt-to-income ratio, and sometimes also their spending habits.
Based on this data, the AI algorithms can assign a credit score to a potential debtor, which lenders can use to make informed decisions about whether to approve a loan and how high the interest should be.
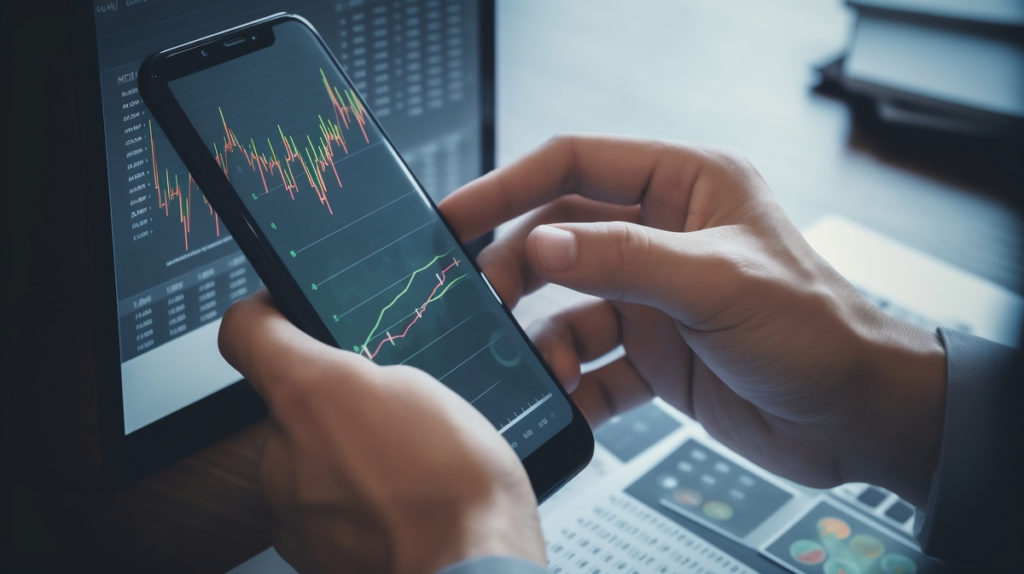
Debt repayment prediction
AI can help debt collection companies predict debt repayment by analysing certain data, for example debtor’s income expenses, any credit score they were given, or payment history. Based on these insights, the AI-powered algorithms can help predict how much of a specific debt or a debt portfolio will be repaid.
AI can also be used to create decision trees for debt collection, which can help financial institutions determine the most effective strategies for collecting debts. The decision trees are powered by machine learning algorithms, which analyse various data to create an optimal decision-making process.
With the decision trees, debt collectors can increase the chance of successfully recovering overdue payments, by taking the best approach for a specific debtor, considering their financial situation and the age of the debt.
Investment risk analysis
Similarly, predictive models can help companies identify potential risks to their investments. The AI models can assess the risk of a particular investment by predicting possible future outcomes based on trends and historical market data. Natural Language Processing methods can be used to analyse news, social media and other sources of information to identify certain sentiments that could indicate that an investment will be a hit or miss.
What is more, AI can help investors analyse their portfolios by identifying the best combination of assets that have the potential to maximise their profits with minimal risk.
AI can also be used to automatically analyse stock market reports. Given their growing number and length, it is worth reaching for solutions that automate the analysis.
Preparing personalised offers thanks to AI-generated insights
Another example is using predictive models to analyse customer data to create personalised offers for a bank’s clients and improve their satisfaction.
AI analyses customer data, including their transaction history, credit score, age, income and other relevant information. Once the data is analysed, the next step is to segment customers according to their financial habits and needs. Based on the identified segments, banks can prepare personalised offers that answer specific needs and preferences. For example, customers who frequently use credit cards may be interested in reward points, cashback, or low-interest rates on credit cards.
AI-supported trading
AI can be implemented to automate trading using trading algorithms trained on historical data from multiple sources. The algorithms analyse large amounts of data, such as market trends, economic news and indicators to reveal patterns in market behaviour and transactions. The program then executes trades automatically.
Automated trading algorithms can analyse market data much faster than humans, almost in real time, which improves efficiency and profitability.
Our team worked on a solution for predicting which shares would gain or lose value. We decided to test the hypothesis put forward by economists Lauren Cohen, Christopher Malloy of Harvard Business School, and Quoc Nguyen of DePaul University, who state that it is possible to predict an increase or decrease in stock share prices of a given company by comparing stock market reports.
The researchers used text analytics methods to spot similarities between the SEC regulatory filings. They proved the hypothesis that companies whose reports are similar year-to-year will gain in the stock market. On the contrary, companies whose reports contain a significant number of differences are more prone to future losses or even bankruptcy.
We downloaded thousands of stock market reports to conduct our analysis using NLP methods and test the hypothesis. Our findings suggested that the hypothesis is true and companies whose reports were very similar achieved a higher profit.
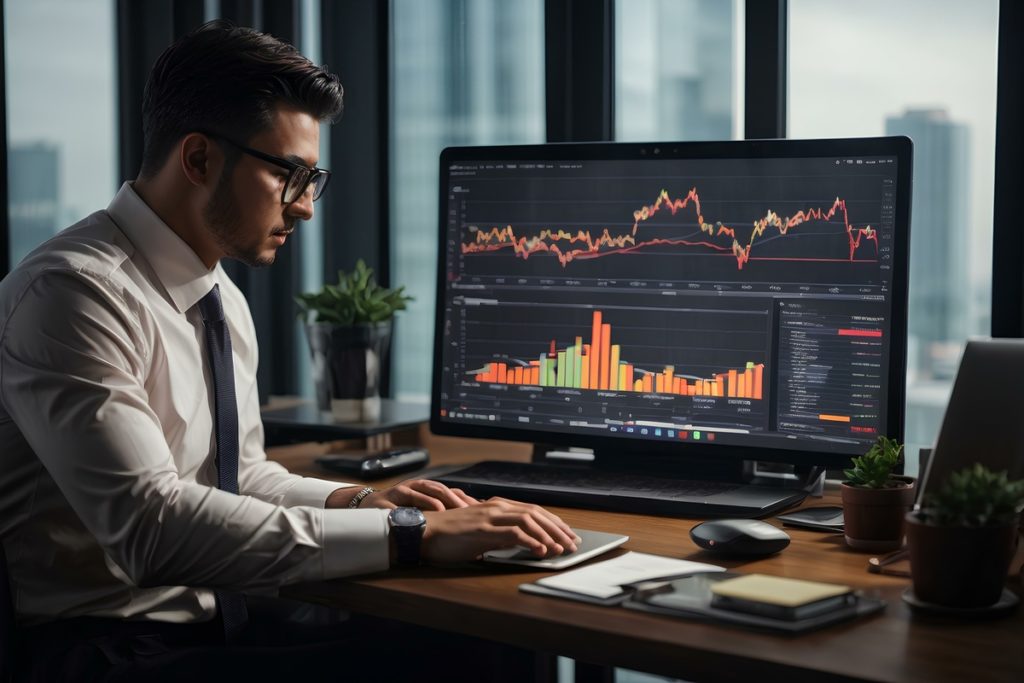
Forecasting market trends
Machine learning algorithms can be used to analyse large amounts of data and make accurate forecasts about financial trends and outcomes. This may help companies make better investment decisions, avoid certain risks and improve customer experience.
Using predictive modelling methods, AI can analyse past market trends and predict future ones based on those insights. Through sentiment analysis of social media posts and other opinion sources, AI can assess public sentiment about a particular service or product. Thanks to the knowledge of how people feel about it, businesses can make better decisions on how to position their products and adjust their strategies accordingly.
AI vs EU regulations
AI, like any tool, may be used for the wrong purposes. Certain measures should be taken to protect people from the adverse effects of misusing AI-powered solutions.
The AI Act is one of the first attempts to do that. It is a proposed European law regulating the use of Artificial Intelligence, banning high-risk applications. It’s predicted that the AI Act may give rise to similar regulations globally.
Take advantage of AI in finance
Modernise your solution using the power of AI. Automate manual tasks, quickly analyse large amounts of data to identify trends, forecast future performance and evaluate potential risks.
Our experts will advise you on how to best apply AI in your specific business case. Contact us via the form below.
About the author
Our blog
Recommended articles
Contact us